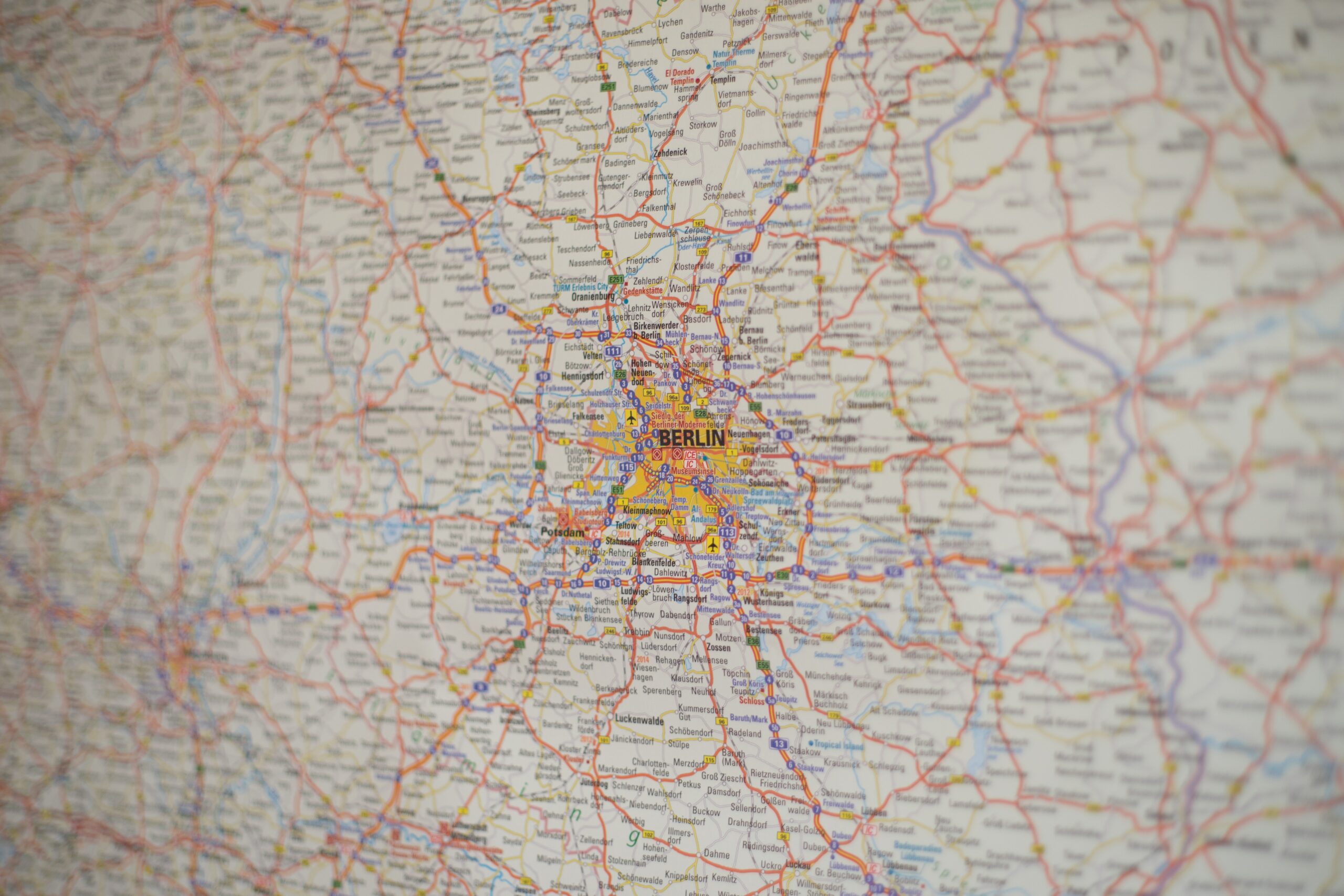
Comprehensive Location Intelligence Across the Globe
How dataplor Differs from Other Location Data Companies
Whether they’re looking to improve site selection, add precision to marketing, or gain insight about the competition, companies of all kinds—from real estate to retail to logistics—are dedicating considerable spend to location intelligence.
As demand for this data continues to rise, supply has followed suit. What’s more, now that the risks of free location data are more widely understood, brands are gravitating towards third-party providers whose data will grant them the granular insights needed to grow at scale without sacrificing accuracy.
But not all providers are created equal. From the collection process to the final product, each offers a different path to location intelligence. Two features that distinguish dataplor from other location data companies are its combined use of AI and human expertise as well as its solution-forward approach to client satisfaction.
A technology-smart process backed by human know-how
By relying on technology and human expertise, dataplor has crafted the most rigorous and global data collection process in the geospatial data business.
Where other providers suffer from an overreliance on human sources and outdated technology (which can lead to errors and inconsistencies), dataplor employs a combination of AI, machine learning, and deep learning for collection. Thanks to our significant investment in these tools, we’re able to use AI call bots to gather up-to-date information, deep learning and image recognition to expand and verify datasets, and machine learning to update attributes and identify issues and patterns in the data.
For example, we might establish a record with a call bot by confirming a restaurant’s phone number and operating hours. From there, we can use image recognition tools to verify on-the-ground information and, if it’s a chain, identify other locations. This enables us to avoid inaccuracies, such as including the record for a location in San Antonio, Texas in a dataset focused on Austin.
Once this data has been collected, dataplor knows precisely when and how to improve it. This is largely thanks to our multipronged approach to enhancement. Before a dataset containing the record above makes its way into a client’s hands, for instance, it will have gone through multiple stages of refinement. These could include dataplor’s custom-built machine learning technology, which eliminates duplicate records (a major issue with POI data), as well as our proprietary enhancement engine, which automatically identifies incorrect geocodes, addresses, and other faulty location attributes.
In addition to leveraging cutting-edge technologies, we know the value of human sources. Our network of over 100 validators does the important work of last-mile verification in more than 200 countries. Each of these local experts has been trained to identify and improve POI records by ensuring that our AI machine learning tools are correctly tagging information. This allows us to offer our customers industry-leading accuracy on an international scale—a veritable rarity in today’s location data market.
A solutions-focused approach to global intelligence
While many companies in the geospatial data market boast a wide array of products and services, dataplor remains focused on places data alone. This enables us to ensure the quality of our product instead of spreading ourselves thin across different paths to location intelligence.
This focus also provides dataplor’s customers with a solutions-focused resource rather than a one-size-fits-all headache. Some providers, for instance, only sell out-of-the-box data products that come as-is, which buyers need to somehow adapt to their specific needs and contexts. Unfortunately, this can lead to wasted hours, unexpected costs, and misleading insights.
By contrast, dataplor offers customers the solutions they need when they need them, such as custom data collection and tailored datasets. To do so, we rely on our POI know-how to identify hard-to-find places or data points; recently, for example, we worked with a major manufacturing company in India to deliver a dataset focused on competitors’ EV charging stations, gas stations, parks, libraries, and other infrastructure complementary to transportation. This helped the client determine how to plan out their EV charging network and gain market share in the electric vehicle space.
Because dataplor deals in POI data alone, we’re able to work with clients across global regions to solve problems and explore opportunities as they arise. Put otherwise, with dataplor, customers can rest assured that they won’t be left guessing with an unwieldy dataset—instead, they’ll be provided with the ongoing support necessary for truly global POI data-driven intelligence.