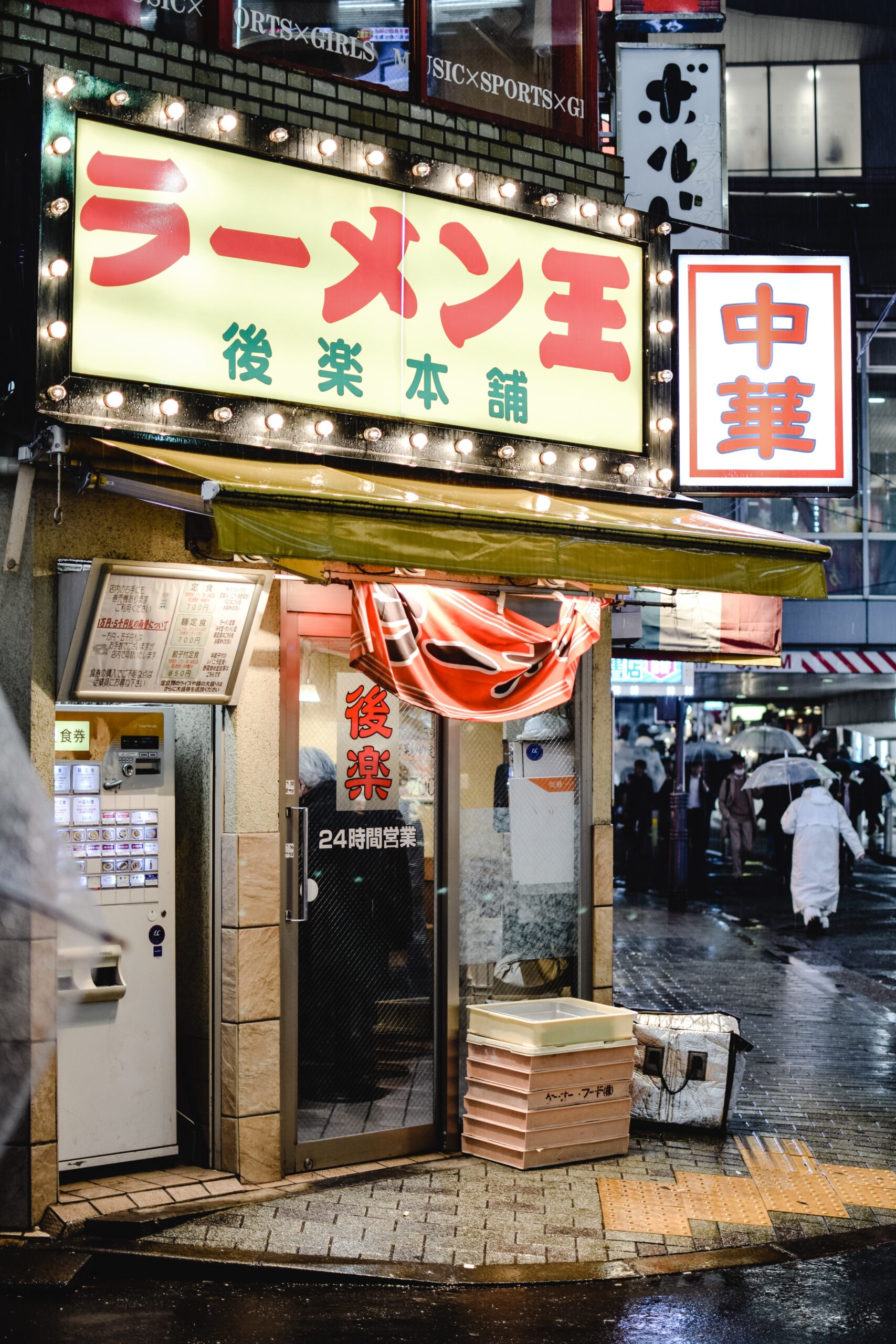
Comprehensive Location Intelligence Across the Globe
How a multifaceted approach to AI drives dataplor’s business
Artificial intelligence is transforming the way location data companies collect, process, and analyze data. With the help of AI, these companies are able to gain a deeper understanding of their customers and the areas in which they operate, which in turn enables them to make more informed and efficient business decisions.
AI’s greatest value is that it can outperform humans in many functions at macro and micro levels. At the macro level, AI can analyze enormous amounts of data quickly, making it possible to find trends and make predictions that humans would miss. At the micro level, it can sift through minute details and eliminate data duplications and other errors that might pass by human eyes.
At the same time, the key to leveraging the power of AI is a multifaceted approach that makes use of its strengths while anticipating and accounting for its weaknesses. Black swans are the classic example. England only has white swans, so an AI trained there would conclude that every swan must be white, and consequently miss on all black ones. This points to a weakness of AI: it only knows what it has been shown, and, unlike humans, it struggles to fill in the gaps between things it does not already know. The consequences can be serious, as in the example of a self-driving vehicle that failed to brake because it identified a pedestrian and their bike as unknown objects.

That’s why dataplor employs a multifaceted approach to AI that maximizes its abilities while avoiding potential drawbacks. Below, we explore three ways in which dataplor’s deployment of AI gives it a competitive edge: drawing on deep image processing and data enhancement; analyzing data at scale to develop predictive models; and leveraging the power of computer-human synthesis.
How dataplor uses AI to analyze images and collect and enhance data
In addition to being worth a thousand words, pictures provide key clues as to when a business opens or closes, what kinds of beverages a restaurant offers, or whether a new location is viable in a developing area. AI systems have given new life to photos because of their capacity to analyze huge amounts of them and identify important information. Called deep image processing, this application lets dataplor draw untapped location intelligence from the multitude of photos publicly available online, photos taken by in-house human validators, and images gleaned from alternative sources and partners like dash cam footage.

Imagine you’re a CPG executive tasked to undertake a global search for restaurants in major cities in need of a soft drink provider. Dataplor’s AI-based deep image processing can pour over photos taken by individuals from inside restaurants in Sydney, Kolkata, Bogotá, or San Antonio, scanning pics of menus, counters, door stickers, soft drink dispensers, and so on, looking for a brand name that signals a provider or lack thereof. A global CPG company can then use this intelligence to identify new business opportunities.
Another application of AI is data collection and enhancement. Dataplor uses AI call bots to get ground signals from across the globe at minimal cost. Language-trained bots call businesses to ask about hours or services, providing detailed local business data at scale. For example, AI callbots can find out a business’ hours or verify whether those hours are correct. In tandem, machine learning systems dig through this data to eliminate duplications, minimizing inaccuracies and rendering the data immediately actionable for a wide range of use cases.
How dataplor uses AI to help businesses predict the future
One of the more promising applications of AI is predictive analytics: the use of historical data, machine learning, and statistical algorithms to identify the likelihood of future outcomes. Location data companies use predictive analytics to forecast future customer behavior and make data-driven decisions. For example, a company can use predictive analytics to identify which neighborhoods are likely to see the most growth in the coming years and invest resources accordingly.
Photos also come into play with predictive analytics, this time as a source of historical data. An AI analysis of storefront photos from one year might reveal lots of construction and development that disappears from photos five years later, signaling a downturn not yet evident in standard economic indicators.

AI can also crunch location data to create predictive models that help businesses make better decisions: for example, helping a retail store to determine the best location to open a new branch or aiding a hotel in predicting seasonal demand for travel accommodations.
In addition, location data companies like dataplor use machine learning to analyze satellite imagery of a particular area to identify patterns in land use, such as the location of buildings, roads, and infrastructure. This can be used to gain insights into population density and land values, which real estate companies can use to identify areas with high potential for development or investment.
How dataplor’s computer-human synthesis gives it a competitive edge
Despite these benefits, AI systems can be biased or make errors, and humans are needed to detect and correct these issues. One way to do this is for humans to provide domain expertise. For example, a human with expertise in location data for the retail industry can help ensure that the AI system is properly analyzing the information it receives and making predictions that are relevant to the industry. An AI might mistake a distressed brick warehouse in the Allston area of Boston as a sign of economic decline, for example, when it’s actually a newly renovated coworking space.
For these reasons, dataplor uses human-computer collaboration to anticipate and improve the performance of its AI systems. Specially trained human validators, with expertise across cultures and languages, act as eyes on the ground and provide real-time feedback that AI systems can use to improve. Validators spot challenges like wordplay in business names or cultural differences in how addresses are formulated and make sure that data is tagged and processed accurately.
For all these reasons, AI will keep growing as a centerpiece of the location data industry. The edge, however, will go to the companies that know how to identify its limits and leverage human-AI collaboration to succeed.